An average human hair is, on average, about 100 microns in diameter. A semiconductor chip, such as those manufactured by Broadcom, in Breinigsville, Pennsylvania, measures about 25 microns–that’s pretty small and difficult to see. Every day, Broadcom makes tens of thousands of these tiny discs, which are used in electrical or photonic devices and circuits. And these infinitesimal items have a complex manufacturing procedure–they are built up in several layers via a sequential process. This complex process–one of the most complex in the manufacturing industry, says Zheng Yao, Principal Research Scientist at the Energy Research Center, Lehigh University, means quality control during manufacturing is essential.
This is where Yao comes in. He has partnered with Broadcom on a research project directed at improving the company’s quality-control practices on the manufacturing production line. Currently this involves manual spot-checks of the semiconductors–an engineer will manually inspect data and microscopic images of the semiconductors produced during the manufacturing process. This, Yao says, is “time consuming, and also very subjective.” It’s time-consuming because if any problems are identified on the manufacturing line, the entire line will be slowed down while decisions are made. And it’s subjective because, Yao says, if two or more quality-control engineers have different thoughts on one product, time can be lost to resolving such issues–several “person-minutes” per issue. As Yao puts it, “Now say you have tens of thousands of this product every minute, every hour, that’s going to be very, very time consuming.”
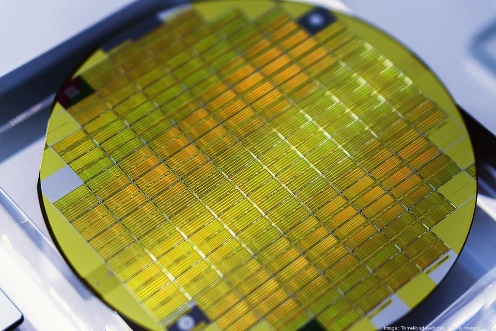
Yao’s innovation is to introduce machine learning to the process. In his project, “Artificial Intelligence for Quality Prediction and Control in Semiconductor Wafer Manufacturing,” funded in part by the Pennsylvania Infrastructure Technology Alliance (PITA) and currently in the model stage at Broadcom, Yao and his research team collect manufacturing data and images supplied by Broadcom. They then develop, via artificial intelligence and machine learning, a set of tools to analyze the data, classify the images, and assess the quality with no interruption to the manufacturing process.
So far, results have been very good. Broadcom is very happy with the research team’s work and has even suggested that the tools be applied on a larger scale. But the team is aiming for higher quality rates on the project, and the graduate student working on the project is also motivated to improve.
Yao’s collaborators in these projects are Yu Yang and Lifang He, both Assistant Professors in the Computer Science and Engineering Department at Lehigh University. Yu Yang specializes in mobile sensing, spatio-temporal machine learning, and reinforcement learning, which allows a bridge between humans and cyber technology. Lifang He studies machine learning/deep learning, data mining, and biomedical informatics, and has been part of a team that won a National Science Foundation award to develop a heterogeneous edge computing platform for real-time scientific machine learning. In addition to this project, the three are also hoping to develop proposals for funding from the Department of Energy and National Science Foundation.
Another project Yao is working on with He also involves machine learning. It’s called “Foundry Unified System for Inspection and Optimization of Networks” or FUSION. In this project, the team is bringing “legacy machines,” or stand-alone devices not connected to a computer or other digital technology, into the Internet of Things era using AI and machine learning. He compares the setup to a washing machine that you might start at a desired water temperature, but something goes wrong: “You have a washing machine, you are washing a silk dress, the temp goes to 200 degrees, but you are doing other things, so you are not aware the temperature has changed, so the silk will get messed up.” Without a warning, the process, and product, can go awry. And at scale, the problem becomes more challenging: “Now imagine you have 100 machines you need to manually monitor,” He says. The FUSION system is currently in the conceptualization stage and will be lab and fab tested next year.
Some companies may have complex option menus for their tools and thus need to rely on more layers of protection other than a single operator trying to monitor many screens. FUSION utilizes both machine learning and image analysis algorithms to refine images taken during the manufacturing process, fine-tuning the algorithms specifically for their needs. They use object detection (“this is a cell phone” versus “this is a cup,” for example) to capture images from the tool, then enhance the images so that data and information can be extracted. This enables real-time data analysis, allowing deeper insight into the manufacturing process. In this project, the team needs to be able to discern further detail–“the color, the letter, the symbol, everything,” Yao says. And the algorithm has to work with less-than-ideal images, such those of machines located in dark corners. FUSION allows monitoring of multiple tools, so that any legacy machines can continue to be utilized. This can save companies the immediate need to upgrade while also allowing improved performance, record-keeping, and easier data analysis.
The team also wants to help the graduate students on these projects apply classroom learning to real-world applications. Many of the tasks the students perform on the research project are related to concepts they’ve learned in class, Yao says. In the classroom, which he calls a “closed system,” the homework is designed for learning–it will always have a known answer, for example. But in the research environment, the students need to apply their own thinking and research to similar problems, a situation where the problem-solving process comes alive on its own. Yao says, “We are helping the students to develop a way of thinking and a way of exploring by themselves in the future.”
Part of the team’s work with Broadcom involves regular meetings with the company’s engineers. Word of the preliminary success has gotten out, Yao says, and more Broadcom engineers are joining the meetings to ask for help with other issues. This could lead to more developments in machine learning’s role in manufacturing. But in the end, the team will be happy to see this work put to use.
As semiconductors become ever more ubiquitous in our world, everyone will benefit from the fruits of this quality control research. Although this research is not yet in the final stages, Yao sees numerous possibilities for the application of machine learning and AI to manufacturing.